In statistics, the parameter “k” can refer to a variety of different parameters depending on the context. One common context is clustering algorithms, where “k” represents the number of clusters. The choice of “k” is a hyperparameter that needs to be specified before running the algorithm, and selecting an appropriate value can be challenging.
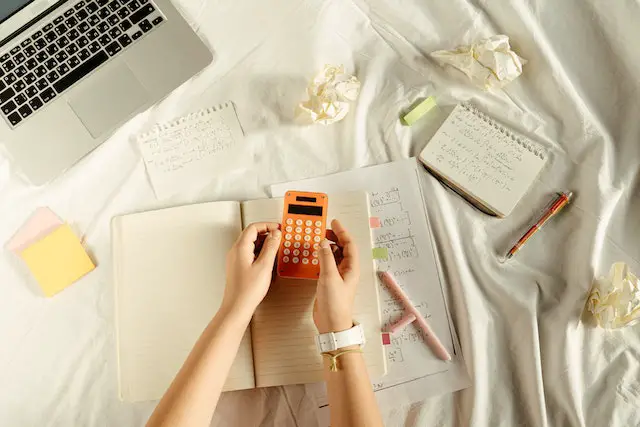
There are several methods for selecting the optimal value of “k”. One commonly used technique is the elbow method, which looks for the “elbow” point in a plot of the within-cluster sum of squares (WSS) versus the number of clusters. The WSS is defined as the sum of the squared distances between each data point and its closest centroid. As the number of clusters increases, the WSS generally decreases, but at some point, adding more clusters will not lead to a significant reduction in WSS. The elbow point is the number of clusters at which the rate of WSS reduction sharply decreases, forming an elbow-like curve in the plot.
Another method is the silhouette method, which is a more objective measure of clustering quality based on the concept of silhouette width. The silhouette width measures how similar a data point is to its own cluster compared to other clusters. It ranges from -1 to +1, with higher values indicating better clustering. To use the silhouette method, we first run the clustering algorithm for a range of k values, compute the average silhouette width across all data points, plot the average silhouette width against k, and look for the maximum value. The value of k corresponding to the maximum silhouette width is considered the optimal number of clusters.
A third method is the gap statistic method, which is another objective measure of clustering quality based on the concept of the gap statistic. The gap statistic compares the WSS of the clustering algorithm on the original data to the WSS of the same algorithm applied to a null reference dataset. The idea is that a good clustering algorithm should have a lower WSS on the original data than on the null data. The gap statistic measures the difference between the two WSS values, normalized by the standard deviation of the null WSS values.
Each of these methods has its strengths and weaknesses, and the choice of method depends on the nature of the data and the goals of the analysis. In practice, it is common to use a combination of methods and to visually inspect the results to determine the optimal value of k.
In conclusion, selecting an appropriate value of “k” is an important step in clustering analysis. There are several methods available to determine the optimal value, including the elbow method, the silhouette method, and the gap statistic method. The choice of method depends on the nature of the data and the goals of the analysis. It is important to note that these methods are not foolproof and that the optimal value of “k” may not be well-defined, especially if the data is noisy or the clustering structure is complex. Therefore, it is essential to use a combination of methods and to interpret the results carefully.